Due to their high energy and power density, lithium-ion batteries are currently the preferred energy storage technology for electric vehicles. However, their properties change in the course of a vehicle’s life: aging reduces the storage capacity and the maximum retrievable performance. These effects are also familiar from other applications, such as smartphones or notebooks. They are the result of the chemical processes in the batteries and cannot be entirely avoided.
For drivers of electric vehicles, the current state of the battery is important information. “With that information, the remaining range at a certain state of charge can be estimated very precisely,” explains Dr. Joachim Schaper, AI expert at Porsche Engineering.
“Vehicle manufacturers can also derive important information from the aging of the batteries, for example the maximum power that can be called up or technical issues that require a visit to the workshop.” The objective is to find a method that provides the most reliable information possible about the condition of a battery.
The most important indicator of this is its internal resistance. Due to the irreversible changes within the energy storage system, it rises continuously during the battery’s lifetime, so the current value is a good indicator of the battery’s "state of health”. However, it cannot be determined directly and must be calculated from different measured values. For this purpose, the batteries are outfitted with sensors that regularly determine the current intensity, the voltage of individual battery cells, and the temperature of cell groups at short intervals.
Their measured values are used to calculate the current state of the battery with the help of an equivalent electrical circuit. The behaviour of the energy storage system is modelled by the internal resistance and battery capacity as well as two combinations of resistors and capacitors (RC elements). “While driving, the power consumption changes again and again, for example when the driver steps on the accelerator pedal. This leads to jumps in the measured values of current and voltage. From these jumps we can calculate the internal resistance of the battery,” explains Jiří Valtr of Porsche Engineering Prague. “The calculation is straightforward: the higher the voltage jumps due to a given current jump, the higher the internal resistance.”
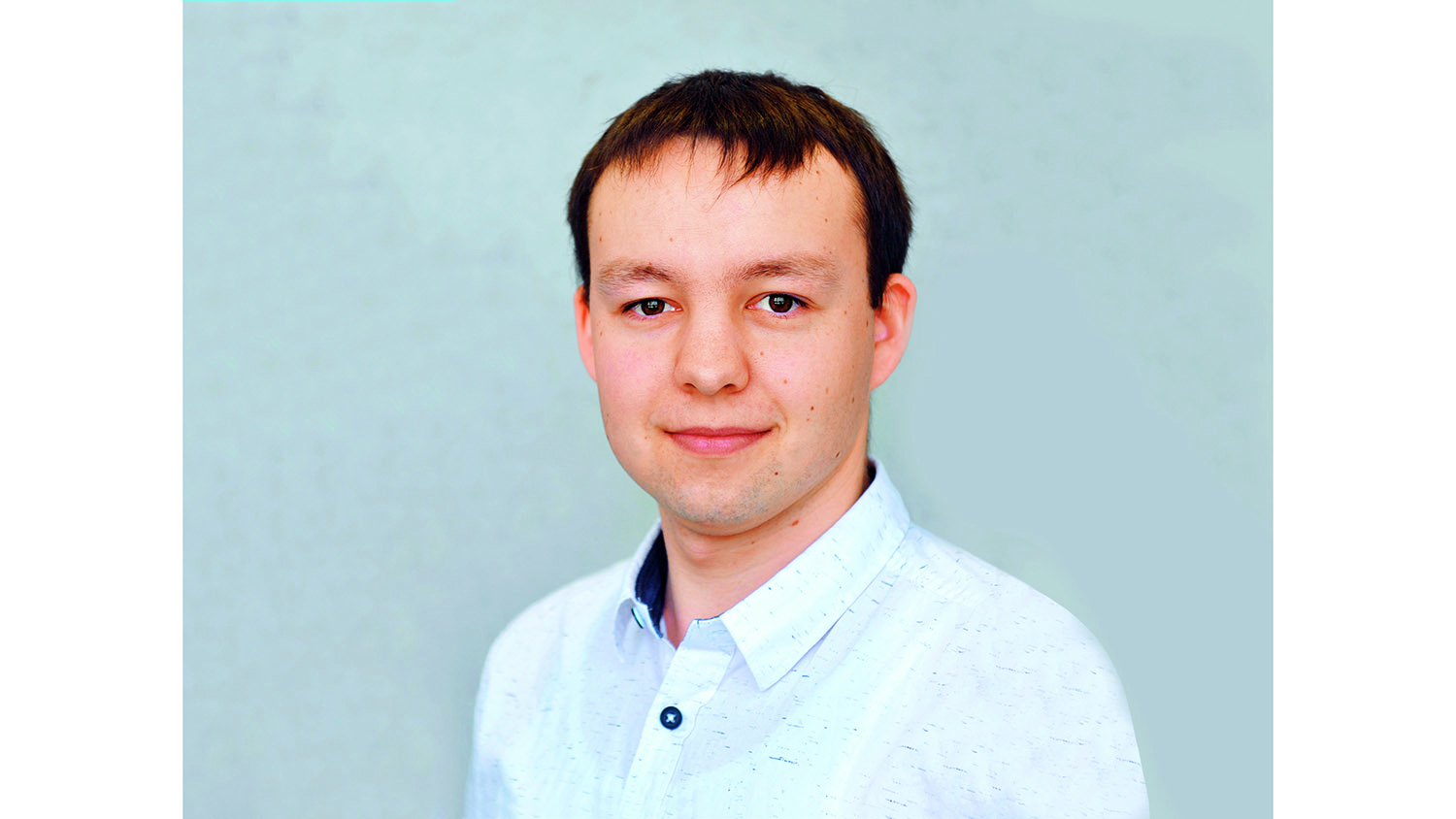
However, the conventional method does have some disadvantages: the values of the resistors and capacitors in the equivalent circuit diagram are not constants, but depend on the temperature and state of charge (SoC) of the battery. And even when all these influences are taken into account, the model still does not represent the actual state of the battery precisely enough. “One reason for this is nonlinearities at low temperatures. When these occur, the battery does not behave like a conventional electrical resistance,” explains Valtr. “That’s why we wanted to replace the conventional model with a more advanced approach to determining internal resistance.” He’s part of a team that has been developing battery management software at Porsche Engineering Prague since 2012. The experts were able to base their work on in-depth knowledge and years of experience in the development of series software used by the entire VW Group.
The developers are relying on artificial intelligence (AI) for this. First they measure the internal resistance of the battery under certain boundary conditions on the test bench. In addition, a correction factor is determined during real trips with machine learning, which makes the calculation of the internal resistance of the battery much more precise by taking into account external influences such as temperature and state of charge, among other things. The data used to calculate the correction factor comes from various sources.
“We wanted to replace the conventional model with a more advanced approach to determining the internal resistance.” Jiří Valtr, Porsche Engineering Prague
One of them is long-term test bench measurements of the internal resistance under different environmental conditions such as temperature, driving cycle and charging behavior. Further valuable input is provided by data from a fleet of test vehicles. “All this data also includes influences that we were previously unable to take into account,” says Valtr. "This includes the outside temperature, the number of charging and discharging cycles already carried out, and driving style, among other things."
More precise forecasting of the remaining range
The new method provides vehicle owners with more precise forecasting of remaining range. The system learns more and more over time and can, for example, increasingly take the owner’s personal driving style into account. It also enables a charging strategy that is adapted to the battery’s state of health: if the battery is already showing clear signs of aging, it can be protected by charging it very gently. The new method is tailored to the limited computing capacity of control units in vehicles and a patent application has been filed.
AI can also take into account influences such as temperature or driving style that have not yet been included in the calculation of the internal resistance.
Even more comprehensive information about the state of health of the battery can be obtained by evaluations in the cloud, because here the computing capacity is practically unlimited. Vehicle manufacturers can, for example, evaluate temperature curves in the batteries and use them to determine the condition of entire vehicle fleets. “From these analyses, the OEMs can identify new relationships,” says Schaper. “Among other things, the question of which factors determine the aging of a battery is interesting. One factor might be the customer’s driving style, but it can also be due to problems with the charging software or the cell supplier.” The ultimate goal is to use the data analyses to further improve future electric vehicles.
The new approach to calculating the internal resistance of batteries is already well advanced. Developers can already create models for the aging process using machine learning from test bench data, data from test vehicles, and log files from customer vehicles. The cloud solution is currently in the works. Customers should benefit from the new range calculation function in about two years’ time, and it will then be gradually integrated into various vehicle models.
Aging processes in batteries
When batteries age, two effects overlap: calendar and cyclical aging. Calendar aging does not depend on use and represents an inherent limit on the possible service life of the energy storage device – even without load. It depends primarily on the state of charge (SoC) and the storage temperature.
The higher the battery is charged, the faster its capacity decreases. High temperatures also accelerate calendar aging. An increase in temperature of 10 kelvins approximately doubles the rate of aging of its electrochemical components.
When operating batteries, the calendar aging process is additionally overlaid by the cyclic aging. The depth of discharge (DoD) plays an important role here. It indicates the ratio of used energy to the total battery energy. A DoD of 80 per cent, for example, means that only 80 per cent of the total energy is used.
The higher the DoD, the faster the battery ages. The state of charge limits must also be considered. A DoD of 80 per cent can be achieved by charging 20 per cent SoC to 100 per cent SoC.
But even a charge of 10 per cent to 90 per cent SoC results in a DoD of 80 per cent. In general, a higher SoC leads to greater cyclic aging.
Current rates (C-rates) also have a major influence on cyclic aging. High C rates and thus high currents accelerate cyclic aging, although high charging currents accelerate cyclic aging much faster than high discharge currents.
During rapid charging at high currents, unwanted secondary chemical reactions can be set in motion in the battery cells: metallic lithium can deposit on the anode surface (lithium plating). The metallic lithium reacts with the electrolyte and forms new cover layers, which consumes further lithium ions. This means that fewer lithium ions are available for energy storage, which leads to an irreversible loss of capacity. In order to avoid such secondary reactions, special fast charging profiles are developed that are precisely adapted to the respective battery cell.
Info
Text: Christian Buck
Contributors: Jiří Valtr, Dr. Joachim Schaper
Text first published in the Magazine Porsche Engineering, issue 1/2020