Equipped to communicate with each other, connected vehicles will exchange information in milliseconds, thereby improving traffic flow and reducing the overall number of accidents.
Offline-cars won’t disappear completely from the streets. In fact, it will be some time before the majority of cars navigate completely independently through traffic. However, vehicles equipped with sensors and cameras already collect and utilize information on a large scale. The amount of data will increase tremendously in the near future.
Porsche and Big Data, a software revolution, and Silicon Valley
Data acquisition, interpretation, and processing play an increasingly important role in the automotive industry. Artificial intelligence and big data will allow us to make better design decisions and build better cars faster. Recently, I spoke with Torben Gräber, Ph.D. student and engineer at Porsche, about data-driven development, a software revolution and Silicon Valley. He shared some exciting insights from his experiences at the Volkswagen Electronic Research Center in Palo Alto, California, where he spent three months working on a local project. You can read about how big data is transforming the way Porsche designs and builds cars below.
Uwe: Torben, your research focuses on applying machine learning methods to vehicle engineering and design. Over the last two years, Porsche has begun to deploy artificial intelligence in numerous pilot projects. We’ve learned quite a bit about the adoption and development of AI solutions. Some of these technologies have already found their way into our cars. What does data-driven development mean to you?
Torben: Collecting and curating data is time-consuming and expensive. But large, high-quality datasets, such as those measured on the road with our Porsche test vehicles, are essential for the efficient use of machine learning models. Our research findings feed directly into our design and development processes.
“Today, advanced electro-mechanical control systems provide precise and responsive steering and optimize braking performance on wet and slippery surfaces." Torben Gräber
Typically, we use mathematical functions and so-called analytical models describing physical parameters to model vehicle functions like steering and braking. Today, advanced electro-mechanical control systems provide precise and responsive steering and optimize braking performance on wet and slippery surfaces.
The conventional mathematical-analytical approaches are increasingly supplemented with artificial intelligence methods. These advances enable us to better model and predict human behavior and to develop intelligent decision-making and control systems, to name but two examples. To describe traffic situations and vehicle behavior fully, comprehensive and accurate data is needed. We, therefore, look at real-world vehicle behavior and utilize measured data and video sequences. That, in a nutshell, is what is called data-driven development.
Data-driven development is key
Uwe: Are we concerned here with a lasting trend in the development of vehicle systems?
Torben: Yes, I think so. Machine learning helps us develop new and advanced technology solutions that improve vehicle safety, comfort and performance. A growing number of carmakers, particularly those that are new to the market, are turning to data-driven development. What the new competitors lack in automotive experience, they make up for in innovative use of technology. These companies often come to the table with something completely new and different. Tesla has shown what possibilities there are. Recently, at Tesla Autonomy Day, the electric vehicle-maker disclosed details about its technologies and strategies. The event is thought-provoking and definitely worth checking out!
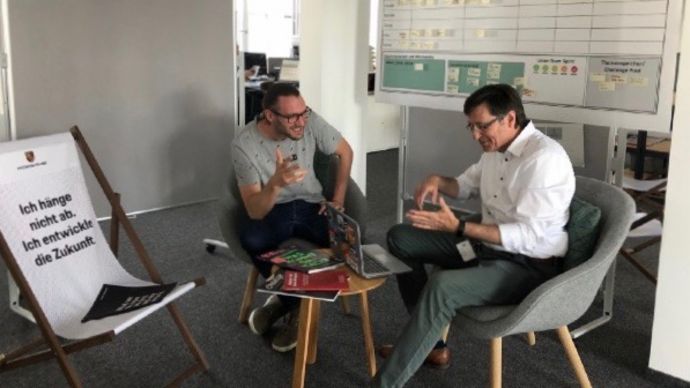
Uwe: Other companies also report on promising projects in this area.Waymo, the former Google self-driving project, is a prime example. The company’s considerable data knowledge is based on extensive data collections from prototype cars and self-driving taxis in California. Other examples are data-based environment recognition for self-propelled vehicles and driver assistance systems. These data-based methods are not only developed by e-mobility startups; they are also supported by the legacy carmakers in the automotive industry. Around the globe, they form alliances with major IT players.
Bit by bit, terabyte by terabyte
Uwe: Torben, how do we make big data accessible and usable?
Torben: An important keyword in this context is “big loop for vehicles.” In the development phase, Porsche uses specially equipped vehicle fleets. During test drives, all internal vehicle data are logged, with additional high-end reference sensors providing exact measurements. We generate sets of data in the terabyte realm each day with our test fleets. Of course, we comply with data protection laws. The focus is clearly on prototype vehicles.
The larger the data storage, the more efficient we can be. The sheer size of the Volkswagen Group offers an opportunity here. We will have access to a wealth of information about all our current vehicles in the field. Our algorithms ensure that only relevant and novel traffic and driving situations are recorded.
"It’s crucial that the developers are able to generate their own application scenarios based on the real traffic data for the functions they have developed." Torben Gräber
The tools are getting more and more complex
Uwe: Do we have to develop our own software for this?
Torben: There are some very good tools that are suitable for vehicle development. Many OEMs and system providers among Tier-1 suppliers are developing in this direction. It’s crucial that the developers are able to generate their own application scenarios based on the real traffic data for the functions they have developed. The measurement results of all vehicles that have passed through these traffic situations must be available at the touch of a button or swipe of a screen.
Uwe: I see it this way: There will be an evolution of tools — similar to the transition from high-level language-based programming to object-oriented programming in the 90s. In the near future, it will no longer be necessary to program every line of code in Python or so. Today, it’s important to find the right IT partners. Our cooperation with universities, service providers and Digital Labs in the Volkswagen Group put us in a good position to meet this challenge.
Is Silicon Valley one step ahead?
Torben: Short distances to our partners are a good thing. But the research activities of the renowned universities and big IT companies and platforms in the US are very advanced. “Big loop” is one of the central research topics in Silicon Valley — in many application areas, not just in the automotive industry.
For example, it deals with the following contents:
- Central data storage, operation of server infrastructures
- Detection of novel use cases for intelligent data collections
- Ultra-fast data collection without expensive hardware in the vehicle
Uwe: How does Germany compare in terms of science and research? Using the experience that we have gained in Silicon Valley, we carry out targeted research assignments at Porsche’s local Volkswagen Group facilities. We exchange and train employees, too.
Torben: What we lack is not the know-how! The “intelligence”, which used to be put into analytical algorithms, must also be put into the database for machine learning procedures. This also means that the value creation is determined by the person who has legal access to the data! And yes, of course, it must also be cost-efficient.
"In any case, the next generations of vehicles will be characterized by a multitude of intelligent functions!" Torben Gräber
There is no way around machine learning
Uwe: Torben, do you see any other relevant use cases besides the much-discussed applications for automated driving?
Torben: In contrast to our colleagues in chassis development, our focus is not exclusively on automated driving functions. So, Tesla’s video is only of limited relevance to us. Nevertheless, the approach of the Big Loop method can be applied to our mechatronic chassis systems. Driving functions can detect and report abnormalities in the chassis systems in certain driving situations. We can then improve the algorithms.
Of course, many chassis functions remain with analytical algorithms. Machine learning procedures are not intended to replace the current practice but to complement it. It’s an additional tool available to us.
Further areas of application are, for instance, predictive maintenance of chassis components and systems or the detection of comfort reducing noises and vibrations around the moving vehicle.
In my opinion, there is no way around machine learning when it comes to recognizing the traffic environment. Its contribution to detecting hazards and obstacles, such as children playing at the edge of the road, is unbeaten here. However, this, in turn, means that large datasets must be available for training purposes. In any case, the next generations of vehicles will be characterized by a multitude of intelligent functions!
Uwe: Thank you very much for the exciting interview and your valuable insights!
Info
Uwe Reuter is Director Resources and Innovation Chassis at Porsche AG.
Torben Gräber is Ph.D. student in Chassis Department and Deep Learning Engineer at Porsche AG. For more about technology and Porsche, get in touch with us on Twitter (Porsche Digital Lab Berlin,Porsche Digital), Instagram (Porsche Digital Lab Berlin, Porsche Digital) and LinkedIn (Porsche Digital Lab Berlin, Porsche Digital).