The Rosetta stone discovered in 1799 was a milestone in the deciphering of Egyptian writings. It contains a priestly decree from the ancient Greek-Macedonian-Ptolemaic dynasty, dated to 196 BC, in three different languages. By a comparison of the texts and characters, it offered a means of deciphering the Egyptian hieroglyphics, which were not decipherable until the 19th century. Since that time, the term ‘Rosetta Stone’ has been used to refer to an essential clue in decryption tasks. Today, AI-based language models, known as large language models (LLMs), are regarded as the Rosetta Stone of the future. “A large language model is based on neural networks and is able to decode the meaning of natural language in context and machine-process it. LLMs can understand, process, and translate language, but also generate new texts,” explains Dr. Joachim Schaper, Senior Manager AI and Big Data at Porsche Engineering.
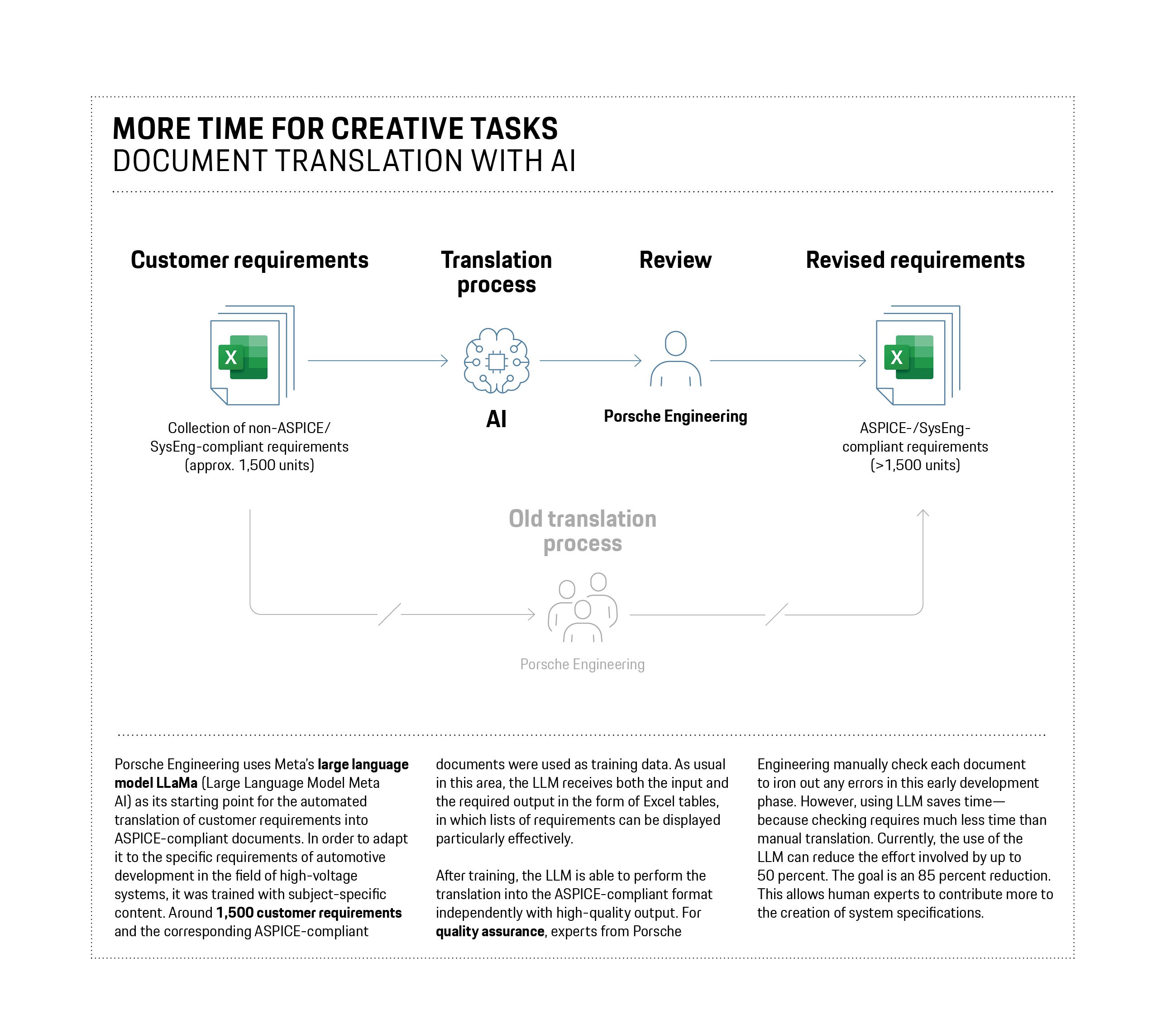
Porsche Engineering uses LLMs to further increase efficiency in the development process. The company uses commercially available LLM tools such as ChatGPT from OpenAI or LLaMa from Meta. “These models are pre-trained by very large amounts of data from the internet and handle tasks such as writing texts on standard topics very well. For use in development, however, we need an LLM that also takes into account our engineering expertise,” says Schaper.
The technical knowledge of Porsche Engineering is taught to the AI using its own data sets from completed development projects. One area of application for LLMs is the revision of customer specifications. Depending on the project, the client, and the development team, their content is written in very different forms. If an existing system is to be technically updated as part of a further development, Porsche Engineering often receives the requirements from existing customer specifications and the scope of the changes from its customers.
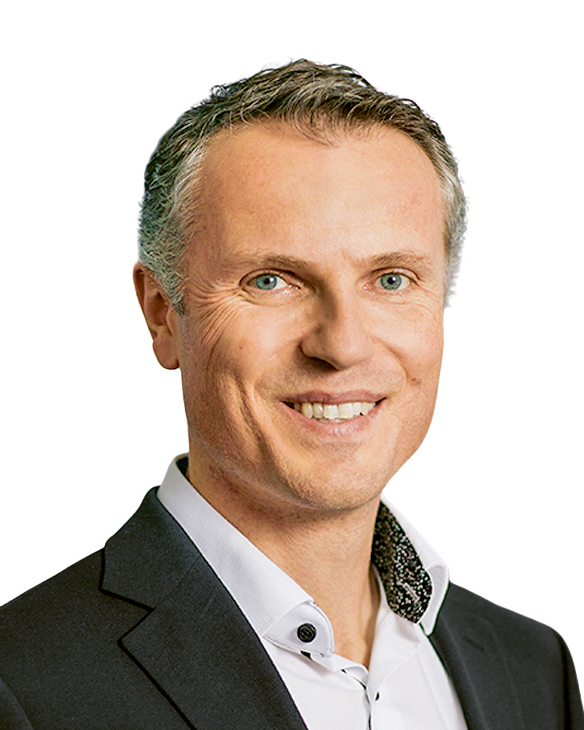
Before the actual development task starts, the developers must completely work through the customer specifications and translate the information contained therein into concrete technical specifications in order to avoid development errors due to ambiguous specifications. Porsche Engineering has recently begun using predefined block templates in the revision of specifications: A basic principle of requirements engineering for the standardized and qualitative creation of requirements. With the aid of this methodology, information is presented in a way that is clear, consistent, verifiable, accurate, and understandable. “Today, our engineers have to do the revision of the specifications as a manual activity. This ties up resources in development and is a monotonous activity for the employees,” says Volker Reber, Senior Manager High-Voltage System Development at Porsche Engineering.
Understanding the context
This task cannot be automated with conventional algorithms. As the formulations in the specifications are frequently not clear, the meaning has to be inferred from the context. Conventional software programs cannot do this intellectual step, but AI can. In the future, LLMs will therefore support the revision of specifications. “As a demonstration project, we revised the requirements catalog for a component of a vehicle,” reports Reber. To train the LLM, a dataset with a few hundred items of information was enough to prepare it for the new task. The model learned how to deal with different semantic forms in the original texts and also learned the text patterns for the output.
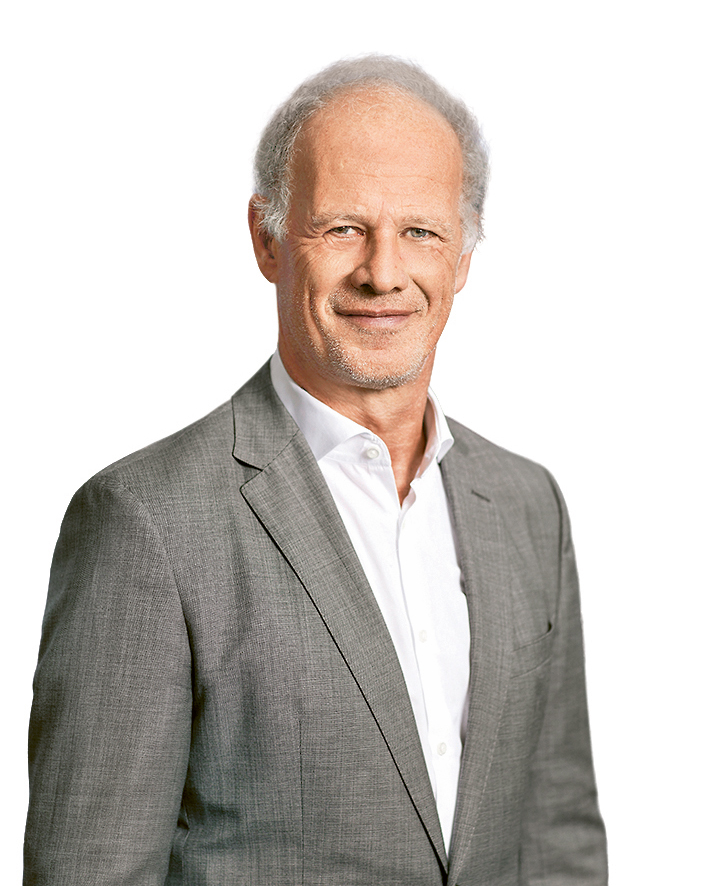
“After this step, the specifications, consisting of a few thousand individual items of information, could be converted to the standard format much faster than through manual processing,” says Reber. As an extension of the project, the trained LLM is used for additional tasks in the field of specifications revision, such as checking for completeness and consistency with regard to the requirements of different systems of the vehicle described therein. The engineers ‘only’ have to check the result produced by the LLM, which means that the workload will decrease over time. “Since the AI is further trained by feedback of the results, the quality of the LLM continuously increases with each project. In the future, it will not only deliver faster, but also much better implementations than a human being could ever do,” Schaper thinks. The LLM has already reduced the effort by around 50 percent during the first test. There are already ideas for further optimizations, which will enable this figure to be improved significantly. Nevertheless, human expertise will still be needed for these tasks in the future.
For Porsche Engineering, the combination of specifically designed and trained AI systems and human expertise has a strategic importance. Many engineering tasks consist of sub-areas that require varying degrees of expertise, experience, and evaluation. Some companies already rely on regions with the best personnel cost structure for certain areas of activity. Porsche Engineering relies on the use of tools such as AI for comparable activities and continues to pursue a strategy of high-level competence among its employees. The experts can then concentrate their valuable working time on the high-competence part of the task. LLMs also offer potential for increasing efficiency in other areas of vehicle development. One example is data management during test drives with new vehicles or systems. If the test drivers detect a malfunction during the tests, they log it and feed it into a central database system.
“Today, we have the challenge that unexpected system reactions are often not recognized as a previously recorded phenomenon and are entered into the system several times,” explains Dr. Fabian Hinder, Lead Engineer at Porsche Engineering. This makes systematic troubleshooting more difficult, as the analysis of database information is associated with considerable manual effort.
Real-time feedback
Much like with the creation of specifications, LLM tools will also take over tasks such as the conversion of the input data into prefabricated semantic patterns and its comparison with existing database entries. The potential of the approach has been demonstrated by a Porsche Engineering team as part of a project in the field of vehicle connectivity. “The test engineer enters the information about their problem into the system during the ongoing tests, and the AI gives real-time feedback on which similar errors are known,” says Hinder. The person then decides whether one of the saved patterns matches their entry or whether to create a new database entry. Errors can therefore be identified throughout the Group and assigned across several vehicle models and platforms. Porsche Engineering is currently conducting another LLM project under the direction of Porsche’s innovation management. Here, too, the goal is to simplify and accelerate everyday work.
“Developers today have to manually query the aggregated data for a problem in the central database. In our lighthouse project, we are creating a concept with which the LLM will carry out this activity in the future,” says Antoon Versteeg, Innovation Manager at Porsche and responsible for the Intelligent Enterprise innovation portfolio. “Unlike the other” LLM applications, however, we are dealing with a very large amount of complex numeric data that needs to be processed and synchronized.”
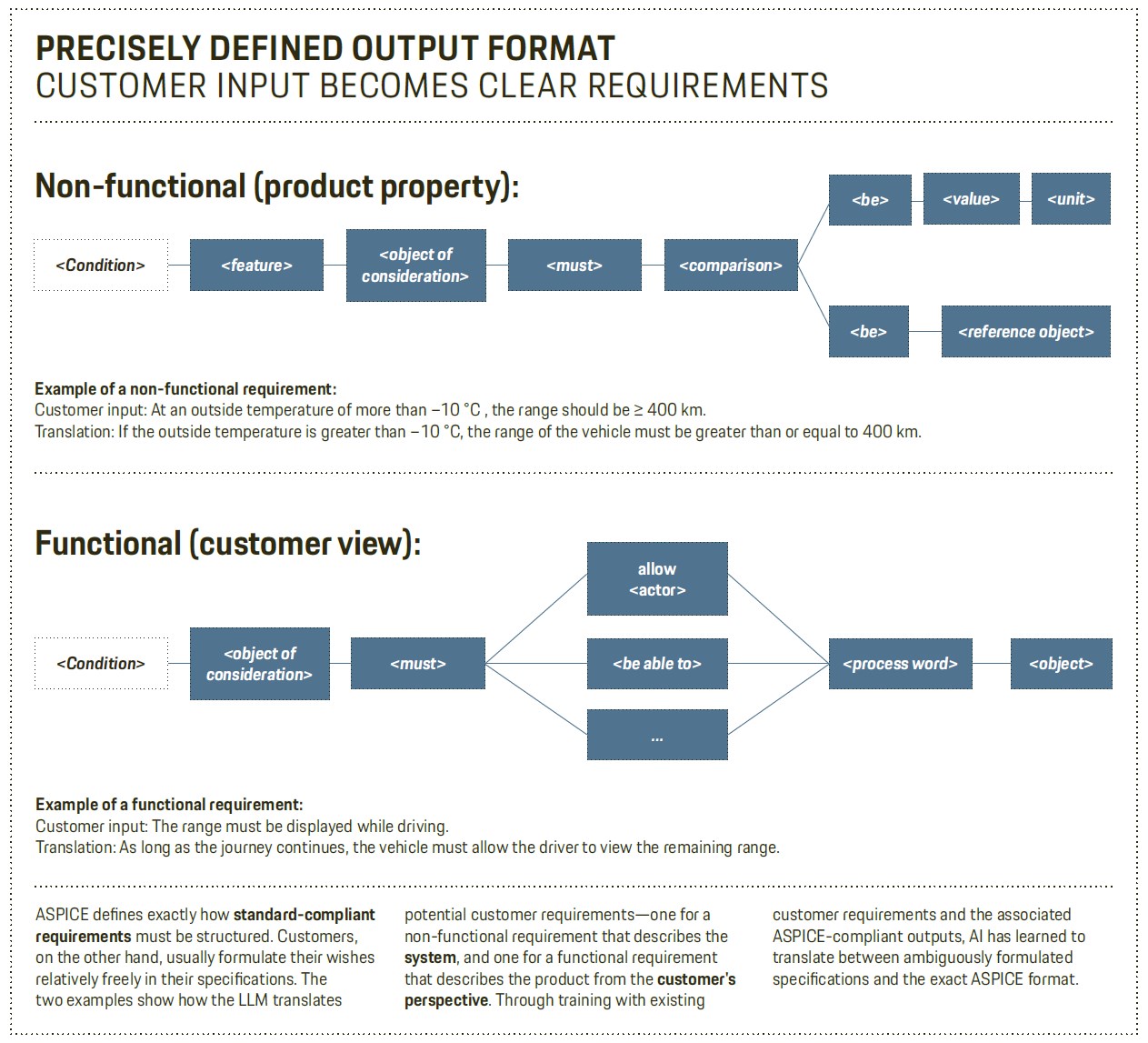
The software developers therefore apply a variety of tricks that combine multiple classic numerical methods and AI. The experts receive results in just a few seconds. “The automotive industry is only just beginning to use LLMs, but thanks to their early adoption in our projects, we are already significantly increasing efficiency in the development process by integrating LLM tools,” says Schaper. “At Porsche AG, data-driven development will be a key success factor for the future. The use of AI can ensure the necessary efficiency in the development process,” says Dr. Bruno Kistner, Manager Data-driven Development at Porsche. “We are already using this very successfully today and are continuously expanding it with our partners such as Porsche Engineering.”
Info
Text first published in the Porsche Engineering Magazine, issue 2/2024.
Text: Richard Backhaus
Copyright: All images, videos and audio files published in this article are subject to copyright. Reproduction in whole or in part is not permitted without the written consent of Dr. Ing. h.c. F. Porsche AG. Please contact newsroom@porsche.com for further information.