A vehicle overtakes and pulls in again leaving too little distance in front of the car behind—at such moments, accidents are often only narrowly avoided. Today, the driver usually prevents the worst by braking or evading.
The autonomously driving vehicle of tomorrow must also be able to react safely at critical moments, which is why Porsche Engineering is already playing through such scenarios intensively in simulations today. When doing so, the engineers specifically increase criticality, for example by reducing the distance between vehicles. “We are building a complete catalog of critical scenarios that enable us to validate driver assistance systems and functions for highly automated driving,” explain Dr. Joachim Schaper, Head of AI and Big Data at Porsche Engineering and Tille Karoline Rupp, Responsible for Simulation at Porsche Engineering.
“We are currently developing a method that will continue to recognize road users even when that category of road user has not been seen for a long time.” Leon Eisemann Doctoral candidate and specialist in image recognition at Porsche Engineering
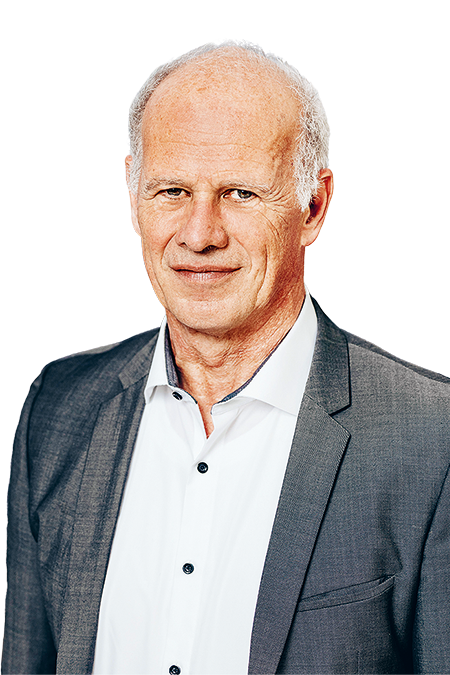
The virtual tests are part of the AVEAS research project (the acronym comes from the German phrase Absicherungsrelevante Verkehrssituationen erheben, analysieren, simulieren, which means ‘collecting, analyzing, simulating traffic situations relevant to validation’), in which, in addition to Porsche Engineering, a further 20 partners are involved, including several Fraunhofer institutes and the Karlsruhe company understand.ai.
Simulatable Scenarios
AVEAS aims to eliminate a major hurdle in the path of autonomous driving: lack of data. In order to validate functions for highly automated driving, billions of test kilometers would theoretically have to be driven. Because this would involve an enormous amount of time and money, real journeys are supplemented by journeys in the simulation. However, this is particularly difficult in critical traffic situations, as there is a lack of real basic data for the simulation— after all, in normal traffic situations, marginal situations rarely occur. AVEAS is intended to fill this gap. The aim of the project is to evaluate test drives automatically and to prepare the critical traffic situations as simulated scenarios.
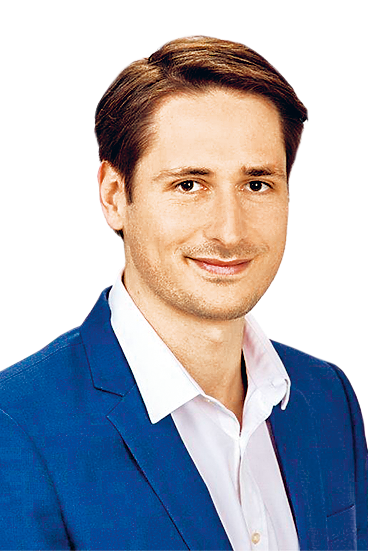
Porsche Engineering is contributing a number of key components to this. Forexample, a JUPITER test vehicle (Joint User Personalized Integrated Testing and Engineering Resource) is being provided for the test drives. It is equipped with cameras, radar, and lidar sensors and sends the data it measures to the cloud. Porsche Engineering also handles the evaluation: Algorithms automatically record the course of the road, the position of other road users, and the road users’ behavior. The machine learning methods used are constantly being refined.
“We are currently developing a method that continues to recognize road users even when that category of road user has not been seen for a long time, for example because they have been hidden behind a truck,” says Leon Eisemann, a doctoral candidate and specialist in image recognition at Porsche Engineering.
The recorded traffic events are stored in standardized file formats such as ASAM OpenDRIVE (logical description of the road network) or ASAM OpenLABEL (objects and the dynamics thereof). AVEAS can therefore also provide input for other projects, such as route modeling. In a second step, algorithms select the critical traffic situations by focusing, for example, on short clearances or unusually intense deceleration forces.
“We are building a complete catalog of critical scenarios that will enable us to validate driver assistance systems and functions for highly auto mated driving.” Dr. Joachim Schaper Head of AI and Big Data at Porsche Engineering and Tille Karoline Rupp Responsible for Simulation at Porsche Engineering
“The relevant degree of criticality always depends on the driving function to be tested later,” emphasizes Nicole Neis, a doctoral candidate in the field of simulation at Porsche Engineering. For example, if Adaptive Cruise Control (ACC) is to be validated, the need for sudden braking before hitting the tail end of a traffic jam would be a relevant critical scenario. Environmental factors can also trigger critical scenarios. For example, when the vehicle approaches the exit from a tunnel, the glare may dazzle the on-board cameras.
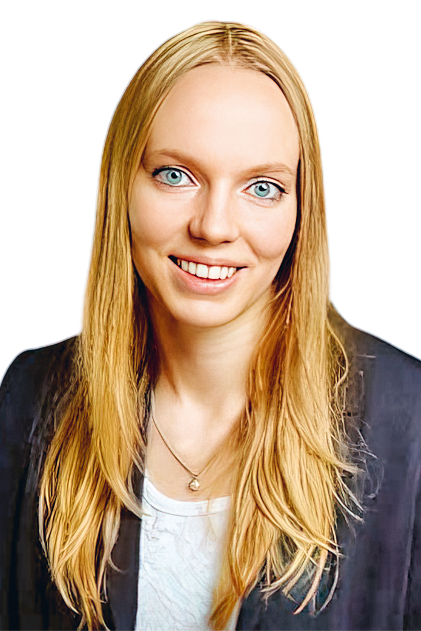
The selection algorithm also highlights those kinds of traffic situations so that they can be used to safeguard driving functions—after all, the autonomous vehicle should react as calmly as a human driver, for example by reducing speed or prioritizing other sensors. First, the (simulatable) driving scenarios consist of position data gleaned from road users over time; experts call this a ‘trajectory-based’ description. In order to be able to vary a driving scenario later on in the simulation, it must be described in abstract terms—i.e. as ‘based on maneuvers’. To this end, meta-processes are derived from the individual trajectories, for example, ‘the vehicle stops at the intersection and then moves off again’. This scenario can then be added to any starting point in a virtual course and can be modified later.
Extension of the test space
The virtual test drives take place in the internally developed simulation environment known as PEVATeC SimFramework (Porsche Engineering Virtual ADAS Testing Center Simulation Framework). The real journey can be reconstructed (simulated) and then be played through after specific modifications have been made, all within the digital world. “In what is known as scenario sampling, the real critical situations are systematically modified, which artificiall extends the virtual validation test space,” explains Rupp.
“The relevant degree of criticality always depends on the driving function to be tested later.” Nicole Neis Doctoral candidate in the field of simulation at Porsche Engineering
A cut-in maneuver, for example, can be repeated thousands of times with different parameters: with a higher cut-in speed, a shorter clearance (time headway), or adverse environmental conditions such as a wet road. This targeted variation of parameters also allows test scenarios to be created that cannot be driven in real terms for safety reasons alone—such as emergency braking to avoid hitting the tail end of a traffic jam.
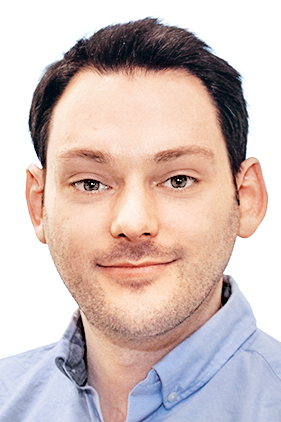
In the last step, synthetic marginal scenarios are used to validate and optimize the driving function in question. To this end, Porsche Engineering is constructing a digital twin of the JUPITER test vehicle. “The ‘Digital JUPITER’ contains the same interfaces and sensors as the real vehicle,” explains David Hermann, a doctoral candidate and specialist project engineer in the field of simulation at Porsche Engineering. “All functions can be tested on a one- to-one basis.” Porsche Engineering will use the Digital JUPITER to evaluate and optimize an Adaptive Cruise Control function and a parking function (Reverse Assist) within the framework of AVEAS.
First Patents Filed
In order for real and virtual test drives to be congruent, a lot of experience is required— much of which must be gained in the analog world. “What is needed is a deep understanding of how real technology and simulations are related,” Schaper emphasizes. A virtual vehicle, for example, has to react to different road surfaces just like the analog model. The project, which was launched in December 2021, has already delivered its first results. “There are many links in the process chain, test drives are underway, and some patents have already been filed,” says Michael Strobelt, who coordinates Porsche Engineering’s participation in AVEAS. However, the fundamental work has also brought challenges. “Mapping reality with its high degree of variability is exacting,” as doctoral candidate Eisemann underscores. Detection algorithms, for example, must be able to identify vehicles from all over the world—not just German ones. “Interface management also plays a major role,” adds Neis, who is also pursuing a doctorate.
Since AVEAS partners contribute data from different sources, the exchange of information requires accurate coordination. The Karlsruhe Institute of Technology, for example, provides aerial photographs of roads, and these are also incorporated into the construction of the digital scenarios. AVEAS will be running until the end of 2024, by which time a scalable pipeline for the evaluation of driving scenarios should be in place—as well as a catalog with many hundreds of thousands of critical scenarios. Both could greatly accelerate development work in the future. Rupp describes the long-term vision as follows: “Scenarios are generated during the test run and used immediately in the simulation to optimize the driving function.” After validation, an over-the-air update could then be used to add the scenarios to the vehicle.
Route Modeling - Precise mapping of the world
Simulations require a world in which road users can move, and with which they can interact. “These route models are a fundamental component of every ADAS/AD simulation—no route model, no virtual test drive,” says Tille Karoline Rupp, Responsible for Simulation at Porsche Engineering. Route models, referred to in technical jargon as the ‘scene’, describe both the driving area (road, parking space) and the surrounding area. They consist of a mathematical model of the road network in OpenDRIVE format and a 3D model that describes the exact appearance and material of the objects. Digital maps, such as those used by commercial navigation systems, are not precise or detailed enough for this. “For example, there is a lack of information about the width of the lanes and road markings,” explains Tobias Watzl, a development engineer in the field of simulation with responsibility for route modeling. Depending on the task, high-resolution maps, freely available OpenStreetMap material, elevation models, and much more can be combined.
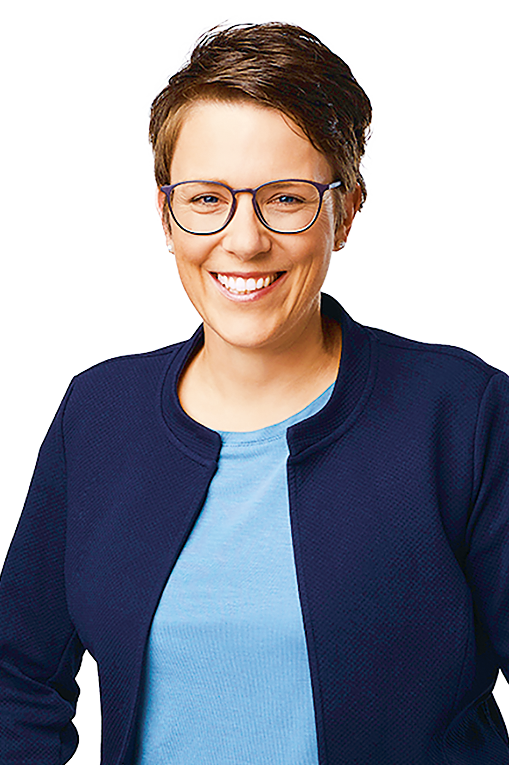
“In order to be able to virtually map the large number of test kilometers required in an appropriate time frame, we are working on our own, highly automated route modeling process,” adds Rupp. The degree of automation in the creation process is constantly increased. As part of the AVEAS project, the JUPITER test vehicles that are used feed measurement data into the route modeling process. They use their lidar sensors to scan the surroundings and transfer the resulting point clouds into the cloud. Since road markings reflect differently from asphalt, they can be easily identified in the lidar data. Special algorithms calculate a continuous overall line from the individual markers (this process even works if individual markers are missing). The result is a precise geo-referenced image of a real street in OpenDRIVE format. This is contrasted with generic route models that are not based on a specific real road (example: ten kilometers of a two-lane freeway). Line modeling requires high precision. The road geometry determined must be accurate down to the centimeter, otherwise, for example, the vehicle distances would not be correct later on when lane changes are simulated. One challenge is that, since roads in OpenDRIVE format are described as mathematical functions, discontinuities can arise that would appear in the simulation as a ‘sharp bend’ in the road. Such discontinuities must be interpolated. “The digital twin often drives a route several hundred thousand times—every detail must be right,” stresses Watzl.
Summary
In the AVEAS research project, Porsche Engineering and its partners are working on automated detection of critical traffic situations in sensor data with the help of AI and storing the situations in a database. They are also varied to generate more test cases. The scenarios are then translated into a simulation, where they are used to validate driver assistance systems and functions for highly automated driving.
Info
Text first published in the Porsche Engineering Magazine, issue 2/2023
Text: Constantin Gillies
Copyright: All images, videos and audio files published in this article are subject to copyright. Reproduction in whole or in part is not permitted without the written consent of Dr. Ing. h.c. F. Porsche AG. Please contact newsroom@porsche.com for further information.