Drivers who are guided by a navigations system will be familiar with a problem: if the lanes of a road are close together, the system cannot recognise which lane the vehicle is in. GPS is not precise enough for this – it can only determine the position to within two to 10 metres – but Porsche Engineering is working on a system that uses artificial intelligence (AI) to calculate a more precise position from GPS data. “This makes it possible, for example, to identify the ideal line on a race track,” says Dr. Joachim Schaper, Senior Manager of Artificial Intelligence and Big Data at Porsche Engineering. The necessary calculations can be performed in the vehicle itself, in a compact computer equipped with graphics processing units (GPUs). “This brings AI functionality to the vehicle,” says Schaper.
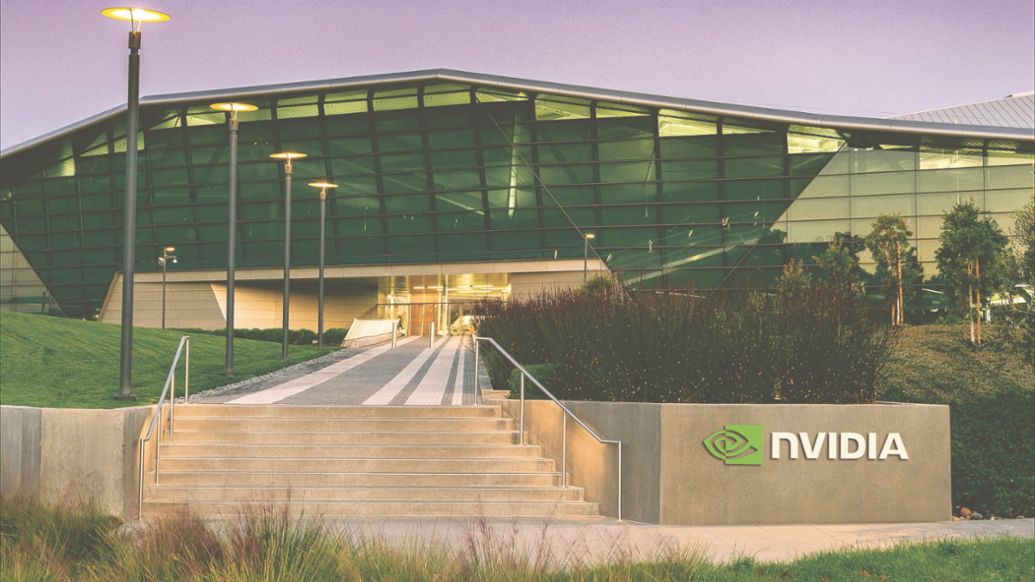
The hardware platform is manufactured by Nvidia, based in Santa Clara, California. “When you hear the name, you don’t necessarily think of the automotive sector,” says Schaper. Most PC users associate Nvidia primarily with graphics cards. Or rather: especially fast graphics cards, such as those required for gaming. This reputation dates back to the early 2000s, when the first games with elaborate 3D graphics came onto the market. Those who wanted to play games like Quake 3 or Far Cry without their screen jerking needed powerful hardware. Among gamers, a favourite quickly crystallised: the GeForce graphics card from Nvidia. It became a best-seller and catapulted the company, founded in 1993, into the top ranks of hardware manufacturers. At the turn of the millennium, the company was turning over three billion US dollars.
AI researchers as new group of customers
In the early 2010s, Nvidia noticed that a completely new group of customers had appeared on the scene who were not interested in computer games: AI researchers. Word had spread in the scientific community that GPUs were perfectly suited for complex calculations in the field of machine learning. If, for example, an AI algorithm is to be trained, GPUs that perform computing steps in a highly parallel fashion are clearly superior to conventional sequential processors (central processing units – or CPUs) and can significantly reduce computing times. GPUs quickly developed into the workhorses of AI research.
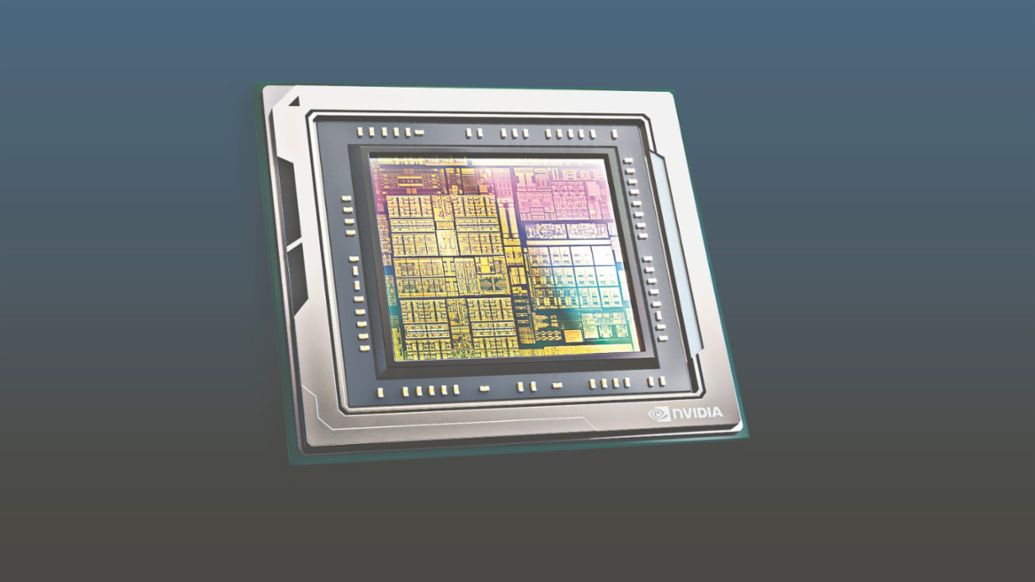
Nvidia recognised the opportunity earlier than the competition and brought the first hardware optimised for AI to the market in 2015. The company immediately focused on the automotive sector: the company’s first computing platform for use in cars was presented under the label Nvidia Drive. The PX 1 was able to process images from 12 connected cameras and simultaneously execute programmes for collision avoidance or driver monitoring. It had the computing power of more than 100 notebooks. Several manufacturers used the platform to bring the first prototypes of autonomous vehicles to the road.
Steady growth in the automotive sector
Initially, Nvidia relied on a pure hardware strategy and supplied the OEMs with processors. Currently, business in the automotive sector has two pillars: cockpit graphics systems and hardware for autonomous or computer-assisted driving. Sales in the automotive sector grew steadily between 2015 and 2020, but still represent a low share of overall sales. Last year, Nvidia’s sales in the automotive sector amounted to 700 million US dollars, which corresponds to a good six percent of total sales; however, sales are increasing by nine percent per year. Jensen Huang, Founder and CEO of Nvidia, sees great market opportunities here. “The cars of tomorrow are rolling AI supercomputers. Only two of the numerous control units will remain: one for autonomous driving and one for the user experience,” he says.
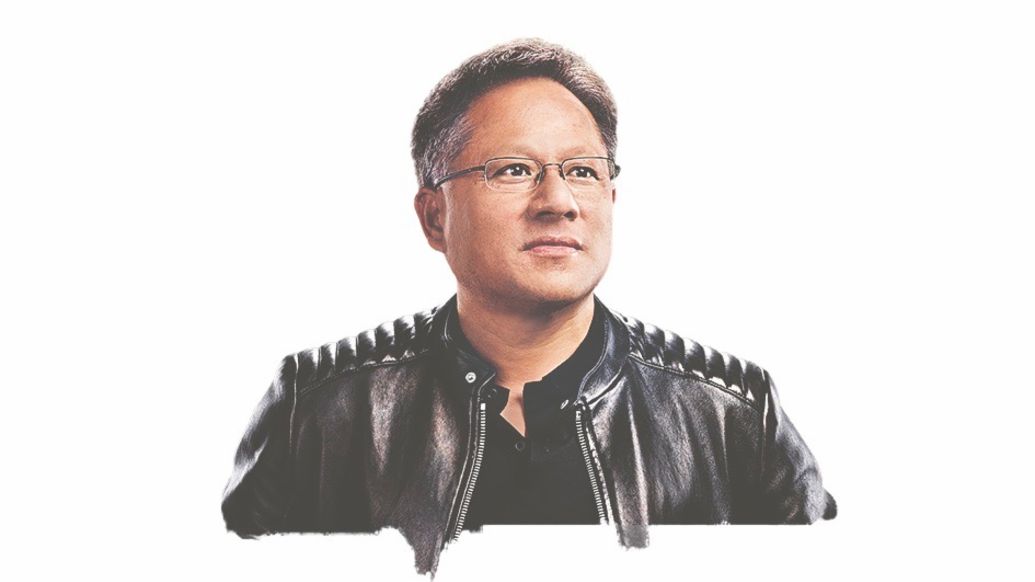
To gain an even stronger foothold in the automotive world, Nvidia has changed its strategy: the company no longer focuses solely on chips, but offers a complete package of hardware and software. “Customers can put together their own solution and save on basic development,” explains Ralf Herrtwich, Senior Director Automotive Software at Nvidia. An OEM that wants to offer a semi-autonomous vehicle, for example, can obtain both the hardware for evaluating the camera images and pre-trained neural networks from Nvidia – for example, one that automatically recognises traffic signs. Unlike other manufacturers, this modular system is open. “All interfaces can be viewed. The OEM can thus adapt the system to its own requirements,” explains Herrtwich. In theory, a manufacturer can use pre-trained neural networks from Nvidia and then combine them with in-house developments.
Nvidia products are System-on-a-Chip
Through this strategy of openness, the American company aims to gain as many OEMs as possible as users, which ultimately also drives the development of the products. “We can best optimise our hardware if we know how it is used,” explains Herrtwich. He offers an example: most Nvidia products are System-on-a-Chip (SoC). This means that a processor is combined with other electronic components on a semiconductor. The automotive sector uses chips with built-in video inputs to which external cameras are connected. But how many inputs are needed? And how should the network connection be designed? Such questions can only be answered in close contact with the users, says Herrtwich. AI expert Schaper has a similar view: “The input from other OEMs is important.” In the current phase, it is crucial to jointly accelerate the development processes.
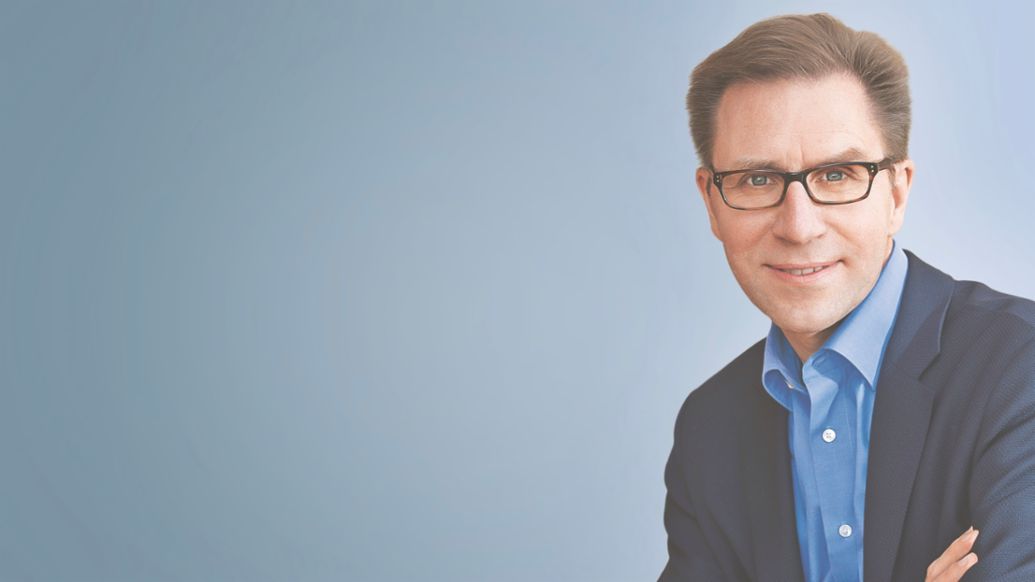
In addition to hardware and software, Nvidia also offers closely cooperating OEMs access to its own infrastructure. For example, manufacturers can collaborate on training neural networks in Nvidia data centres, where thousands of GPUs work in parallel. After all, a self-driving algorithm must first learn to recognise a pedestrian, a tree, or another vehicle. To do this, it is fed millions of images from real traffic on which the corresponding objects have been manually marked. Through trial and error, the algorithm learns to identify them. This process requires a lot of work (such as labelling the objects) and requires high computer capacities. Nvidia handles both. Car manufacturers can thus access an artificial intelligence that has virtually been in school for several years.
Three questions to Ralf Herrtwich
When does artificial intelligence (AI) arrive in the car?
It’s already in the cockpit, for example. Many manufacturers offer voice control based on AI. The performance of these systems has improved noticeably in recent years. In addition, the vehicle is increasingly expected to perceive its environment and react appropriately, as is the case in assistance systems. Here, too, AI is playing a growing role. Such vehicle applications are among the most demanding of all. We expect this area to advance AI as a whole.
When will autonomous driving become a reality?
Robotic vehicles are already being used in localised areas, especially in places where the weather is good. However, for the time being we see the main market for regular vehicles with support functions, that is autonomy levels one to three. Overall, the coming years will be characterised by a competition between such systems – that is by the question of which manufacturer’s system can master most situations. It is less about the aspiration of being completely self-controlled.
How does artificial intelligence change the automotive ecosystem?
The more important software functions become, the more the role of tier one suppliers changes. Their traditionally strong ties to manufacturers are weakening. In the future we can imagine a triangular constellation: OEMs work with technology companies like Nvidia on processors and software modules, and tier one builds the control unit. Some OEMs already attach importance to keeping software functions in their own hands.
Why GPUs are the better AI computers
GPUs are specialised in performing geometric calculations: rotating a body on the screen, zooming in or out. GPUs (graphics processing units) are particularly good at performing the matrix and vector calculations required for this. This is an advantage in the development of neural networks. They are similar to the human brain and consist of several layers in which data is processed and passed on to the next layer. To train them, matrix multiplications are key—in other words, exactly the specialty of GPUs.
In addition, these computer architectures have a lot of memory to store intermediate results and models efficiently. The third strength of GPUs is that they can process several data blocks simultaneously. The processors contain thousands of so-called shader units, each of which is quite simple and slow. However, these computing units can process parallelisable tasks much faster than conventional processors (central processing units, CPUs). When training neural networks, for example, graphics processors reduce the time required by up to 90 per cent.
Info
Text: Constantin Gillies
Contributor: Dr. Joachim Schaper
Text first published in the Porsche Engineering Magazine, issue 1/2021