A freeway, sometime in the early hours of the morning. The sun is low, rushhour traffic is getting heavier. A passenger car is driving in the middle lane and cutting in behind a flat-bed truck on the right. The truck is carrying a vehicle that is positioned backwards on the flat bed with its front facing to the rear. The camera sensors on the car that is cutting in interpret the cargo as an oncoming vehicle, and the emergency braking system intends to intervene. Traffic situations such as these are very rare, but not unheard of. Advanced driver assistance systems (ADAS) must therefore also be trained for these unlikely events. “Rarely occurring cases such as a vehicle transport like this are a classic corner case,” says Arsen Sagoian, Specialist Project Manager in the AI and Big Data division at Porsche Engineering.
The term refers to unusual traffic situations that tend to occur rarely. They also include pedestrians or cyclists on the freeway, lane boundaries that are no longer recognizable because of snow, or roads that, due to their nature, make it difficult for the systems to recognize lane markings clearly.
This is why developers must constantly train driver assistance systems with corner cases in order to further improve them. The problem is that, typically, most of the scenarios recorded on video during normal journeys are similar to each other from a technical point of view and therefore contribute little towards optimizing ADAS systems. Over time, the database of corner cases continues to grow, so that exceptional situations tend to become increasingly rare in new test drives.
The challenge is to identify the remaining unconsidered corner cases in the recordings with as little effort as possible— which is like searching for the proverbial needle in a haystack. A manual search of the video data material is not suitable for largescale use due to the time required and the associated costs.
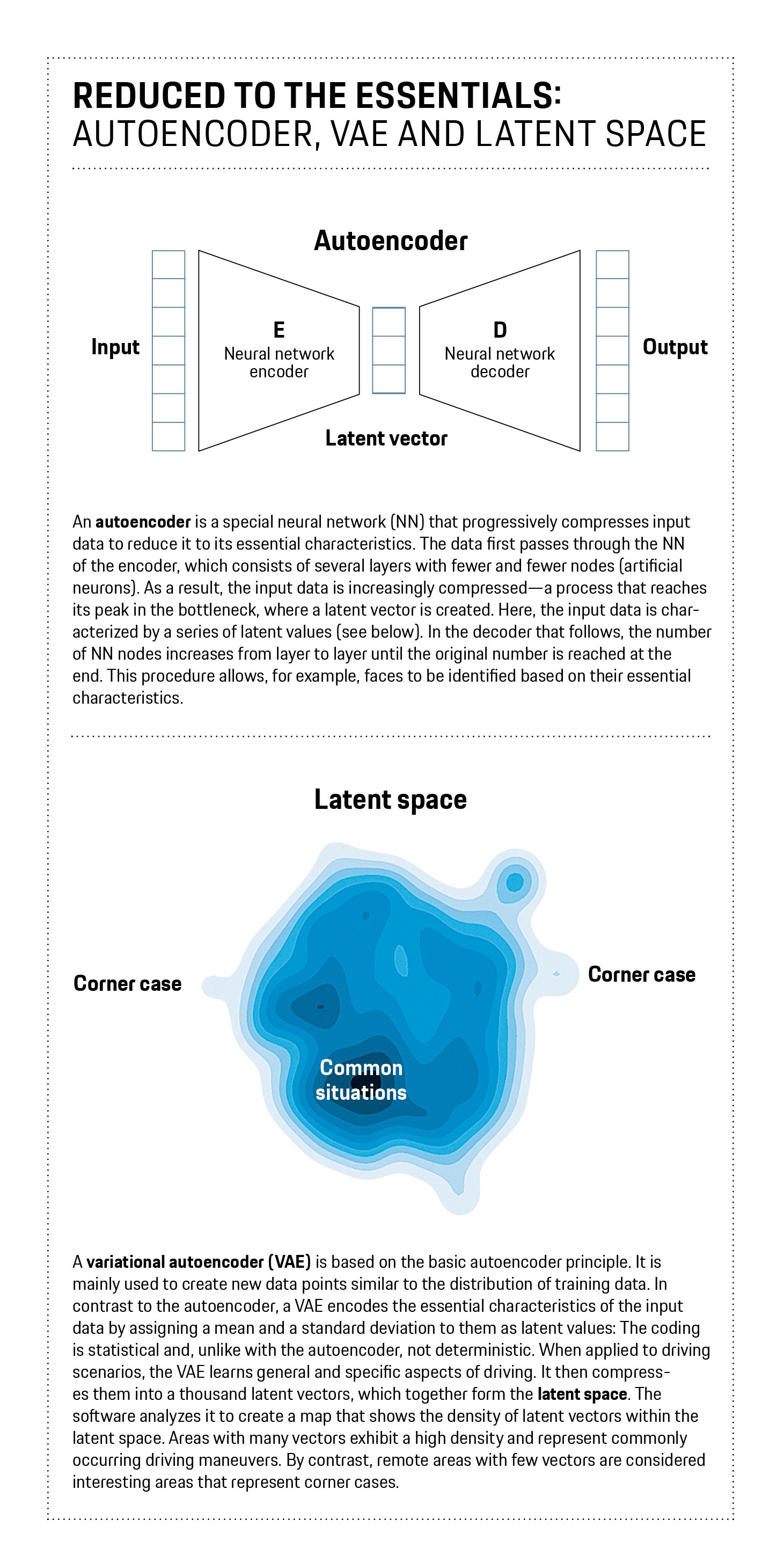
AI finds Corner Cases
In its ‘AI-Based Corner Case Detection’ project, Porsche Engineering instead uses artificial intelligence (AI) methods to automatically search video data or time sequences with pre-processed sensor data and bus signals in order to find the coveted outliers. A variational autoencoder (VAE) analyzes the video and time sequence data recorded during test drives in order to find appropriate situations, their duration, and their timestamp (see box). So far, the method has been used primarily for face recognition. Its deployment in improving ADAS solutions is new. Because VAE is an unsupervised learning process, training data can be created more easily. The runtime is also shorter, allowing the algorithms to be run directly in the vehicle. This means that only relevant data is recorded.
The corner cases collected with the help of AI are then forwarded as new test cases to the teams responsible for functions such as lane recognition. “We had one case, for example, where the edge of some snow on the ground was interpreted within the limits of the system as a lane boundary. This resulted in an uncomfortable lateral maneuver on the part of the vehicle,” explains Daniel Slieter, Tech Lead Verification, Validation & Data Analytics for the driving functions at CARIAD. In the Active Lane Departure Warning (ALDW) system, the data points attached to relevant snow-edge corner cases are spatially highlighted. This allows developers to adapt the functionality of the Lane Departure Warning system to this corner case—with the result that in the event of a comparable scenario within the limits of the system, the driver assistance system will no longer be so easily confused by a snow edge.
AI is far superior to humans in searching for these kinds of scenarios: It takes it only a few minutes to analyze data on around 10,000 kilometers of driving and identify about five corner cases. The quality of the algorithm and the thresholds defined play an important role. Manual evaluation of the recorded kilometers from test drives is reduced to a minimum by the use of AI. “Compared to manual evaluation, we save far more than 99 percent of human working hours thanks to AI, and this trend is rising steeply,” according to Sagoian. “We refer to this as an exponential increase in efficiency, and it allows engineers to concentrate on the actual development of the function.”
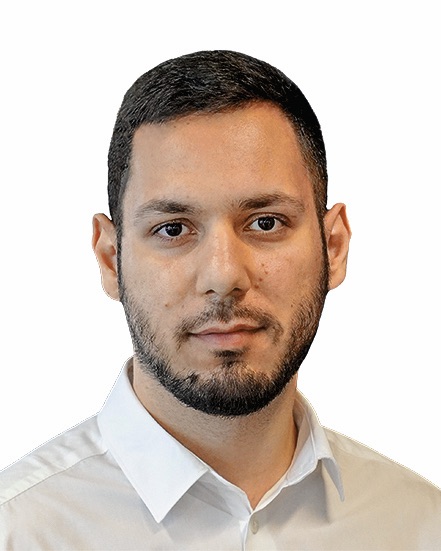
Real-time analysis
For now, all recorded data is loaded into the cloud for analysis. But it doesn’t have to be that way forever: “In the future, corner case detection could be performed in real time during active vehicle operation, because our neural network is a small one that offers good performance,” explains Slieter. “We would then only load the detected corner cases into the cloud instead of the raw data, which would significantly reduce the amount of data transferred this way.” There is another benefit of the new method: AI is not only becoming ever more precise by permanently analyzing data, it also allows for more far-reaching conclusions.
The basis for this is what is known as latent space—an abstract space in which the AI searches the data for patterns and reference images, and thus constantly becomes better (see box). Using this latent space, developers can also identify similarities between countries when allocating corner cases.
In addition, the similarity of new data points to known corner cases can be used to deduce whether a sufficient number of data points has been recorded for validation purposes. “In summary, the VAE looks at the images while the latent space ensures that they are properly analyzed and evaluated,” Slieter explains. The capabilities of the method are currently being tested worldwide. “This allows us to identify very different types of corner cases and also to draw conclusions about the extent to which the results can be transferred from one country to another,” says Sagoian.
The system has identified a particularly large number of corner cases in Sweden and Finland, which is due, among other factors, to exceptional traffic situations caused by snow. Encounters between vehicles and animals are also expected to be more frequent in these countries.
Info
Text first published in the Porsche Engineering Magazine, issue 2/2024.
Text: Claudius Lüder
Copyright: All images, videos and audio files published in this article are subject to copyright. Reproduction in whole or in part is not permitted without the written consent of Dr. Ing. h.c. F. Porsche AG. Please contact newsroom@porsche.com for further information.