Experts regard 2016 as a milestone in the history of artificial intelligence (AI). Largely unnoticed by the general public in Europe and the USA, the computer program AlphaGo competed against the South Korean world-class player Lee Sedol in the board game go and won four of the five games. This was the first time that a computer was dominant in the traditional Asian strategy game. Until then, it had not been possible to teach software the complex strategy of the board game—the required computing power and computing time would have been too great. The turning point came when the AI in the go computer was trained using deep reinforcement learning.
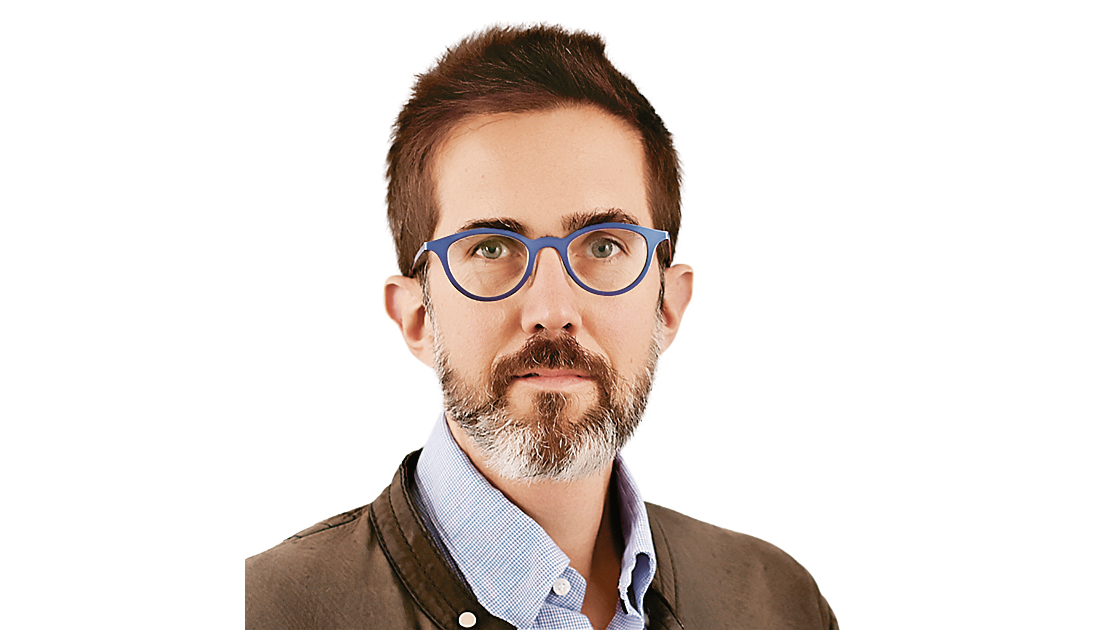
One of the superlative disciplines of AI
Deep reinforcement learning, still a relatively new methodology, is considered one of the supreme disciplines of AI. New, powerful hardware in recent years has made it possible to use it more widely and to gain practical experience in applications. Deep reinforcement learning is a self-learning AI method that combines the classic methods of deep learning with those of reinforcement learning. The basic idea is that the algorithm (known as an “agent” in the jargon) interacts with its environment and is rewarded with bonus points for actions that lead to a good result and penalized with deductions in case of failure. The goal is to receive as many rewards as possible.
To achieve this, the agent develops its own strategy during the training phase. The training template provides the system with start and target parameters for different situations or states. The system initially uses trial and error to search for a way to get from the actual state to the target state. At each step, the system uses a value network to approximate the sum of expected rewards the agent will get from the actual state onwards if it behaves as it is currently behaving. Based on the value network, a second network—known as the policy network—outputs the action probability that will lead to the maximum sum of expected rewards. This then results in its methodology, known as the “policy,” which it applies to other calculations after completing the learning phase.
“PERL is highly flexible because parameters such as the engine design, displacement or charging system have no influence on learning success.” Matteo Skull, Engineer at Porsche Engineering
In contrast to other types of AI, such as supervised learning, in which learning takes place based on pairs of input and output data, or unsupervised learning, which aims at pattern recognition, deep reinforcement learning trains long-term strategies. This is because the system also allows for short-term setbacks if this increases the chances for future success. In the end, even a master of the stature of Sedol had no chance against the computer program AlphaGo, which was trained in this way.
Use in engine calibration
The performance of deep reinforcement learning in a board game gave the experts at Porsche Engineering the idea of using the method for complex calibration tasks in the automotive sector. “Here, too, the best strategy for success is required to achieve optimal system tuning,” says Matteo Skull, Engineer at Porsche Engineering. The result is a completely new calibration approach: Porsche Engineering Reinforcement Learning (PERL). “With the help of Deep Reinforcement Learning, we train the algorithm not only to optimize individual parameters, but to work out the strategy with which it can achieve an optimal overall calibration result for an entire function,” says Skull. “The advantages are the high efficiency of the methodology due to its self-learning capability and its universal applicability to many calibration topics in vehicle development.”
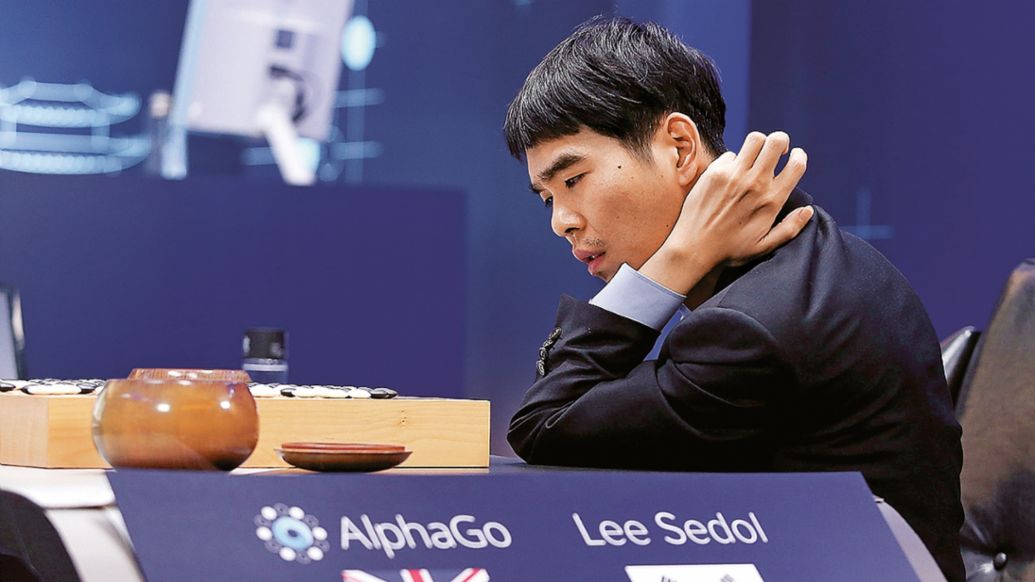
The application of the PERL methodology can basically be divided into two phases: the training phase is followed by real time calibration on engine dyno or in vehicle. As an example, Skull cites the torque model with which the engine management system calculates the current torque at the crankshaft for each operating point. In the training phase, the only input PERL requires is the measurement dataset from an existing project, such as a predecessor engine. “PERL is highly flexible here, because parameters such as engine design, displacement or charging system have no influence on the training success. The only important thing is that both the training and later target calibration use the same control logic so that the algorithm implements the results correctly,” says Skull.
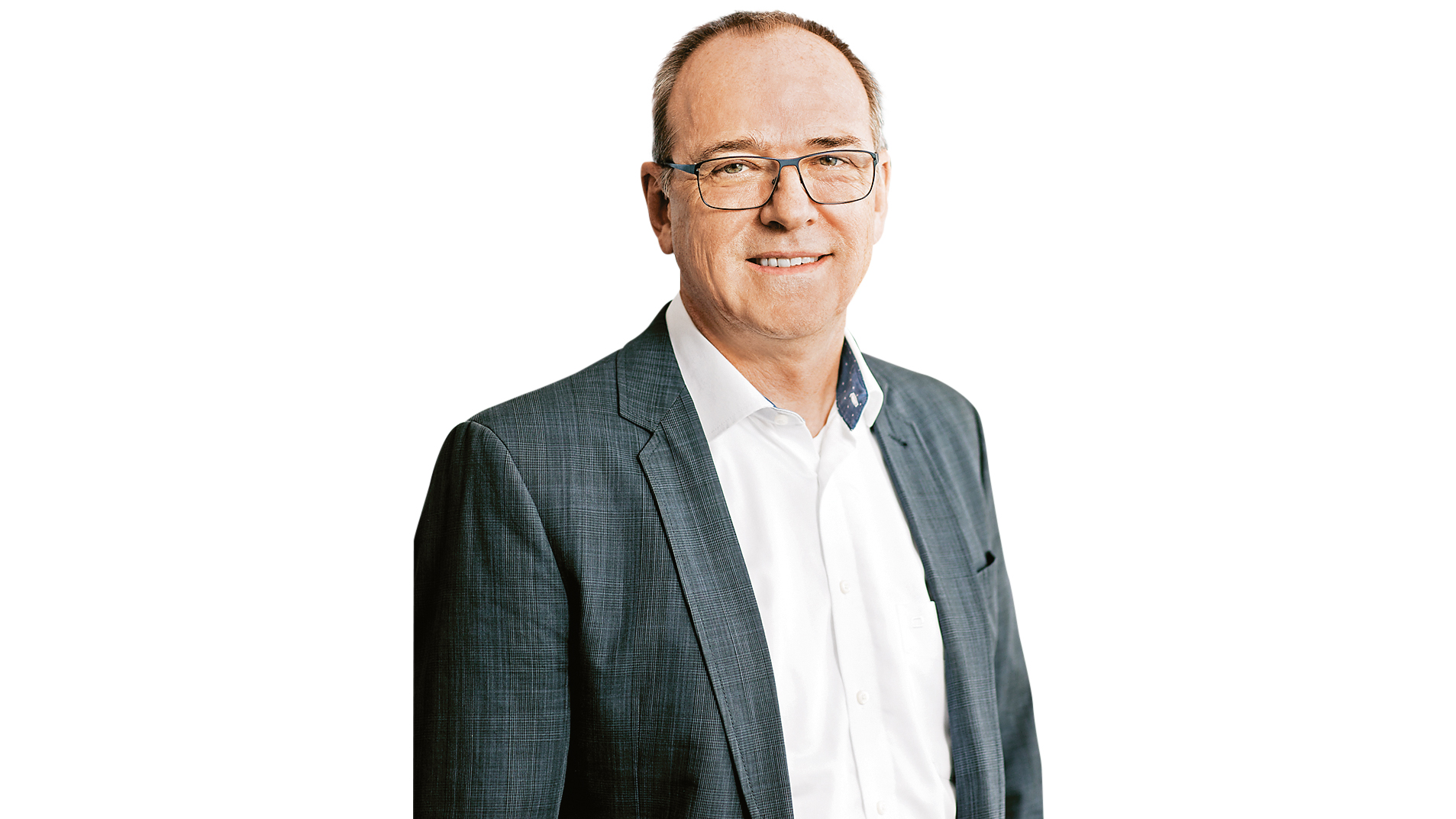
During training, the system learns the optimal calibration methodology for calibrating the given torque model. At critical points in the characteristic map, it compares the calibrated value with the value from the measurement dataset and approximates a value function using neural networks based on the resulting rewards. Using the first neural network, rewards for previously unknown states can be estimated. A second neural network, known as policy network, then predicts which action will probably bring the greatest benefit in a given state.
Continuous verification of the results
On this basis, PERL works out the strategy that will best lead from the actual to the target value. Once training is complete, PERL is ready for the actual calibration task on the engine. During testing, PERL applies under real-time conditions the best calibration policy to the torque model. In the course of the calibration process, the system checks its own results and adjusts them, for example if the parameter variation at one point in the map has repercussions for another.
“In addition, PERL allows us to specify both the calculation accuracy of the torque curve and a smoothing factor for interpolating the values between the calculated interpolation points. In this way, we improve calibration robustness with regards to influences of manufacturing tolerances or wear of engine components over engine lifetime.” explains Dr. Matthias Bach, Senior Manager Engine Calibration and Mechanics at Porsche Engineering.
“With PERL, we improve calibration robustness with regards to the influences of manufacturing tolerances or wear of engine components over engine lifetime.” Dr. Matthias Bach, Senior Manager Engine Calibration and Mechanics
In the future, the performance of PERL should help to cope with the rapidly increasing effort associated with calibration work as one of the greatest challenges in the development of new vehicles. Prof. Michael Bargende, holder of the Chair of Vehicle Drives at the Institute of Automotive Engineering at the University of Stuttgart and Director of the Research Institute of Automotive Engineering and Vehicle Engines Stuttgart (FKFS), explains the problem using the example of the drive system: “The trend towards hybridization and the more demanding exhaust emission tests have led to a further increase in the number of calibration parameters. The diversification of powertrains and markets and the changes in the certification process have also increased the number of calibration that need to be created.” Bargende is convinced of the potential of the new methodology: “Reinforcement learning will be a key factor in engine and powertrain calbrations.”
Significantly reduced calibration effort
With today’s conventional tools, such as model-based calibrations, the automated generation of parameter data—such as the control maps in engine management— is generally not optimal and must be manually revised by the calibration engineer. In addition, every hardware variation in the engine during development makes it necessary to adapt the calibration, even though the software has not changed. The quality and duration of calibration therefore depend heavily on the skill and experience of the calibration engineer.
“The current calibration process involves considerable time and cost. Nowadays, the map-dependent calculation of a single parameter, for example the air charge model, requires a development time of about four to six weeks, combined with high test-bench costs,” said Bach. For the overall calibration of an engine variant, this results in a correspondingly high expenditure of time and money. “With PERL, we can significantly reduce this effort,” says Bach, with an eye to the future.
In brief
The innovative PERL methodology from Porsche Engineering uses deep reinforcement learning to develop optimal strategies for engine calibration (the “policy”). Experts regard the new AI-based approach as a key factor in mastering the increasing complexity in the field of engines and powertrain systems in the future.
Info
Text: Richard Backhaus
Contributors: Matteo Skull, Dr. Matthias Bach
Text first published in the Porsche Engineering Magazine, issue 1/2021